论文阅读笔记:“Empowering Relational Network by Self-Attention Augmented Conditional Random Fields for Group Activity Recognition(eccv 2020)”
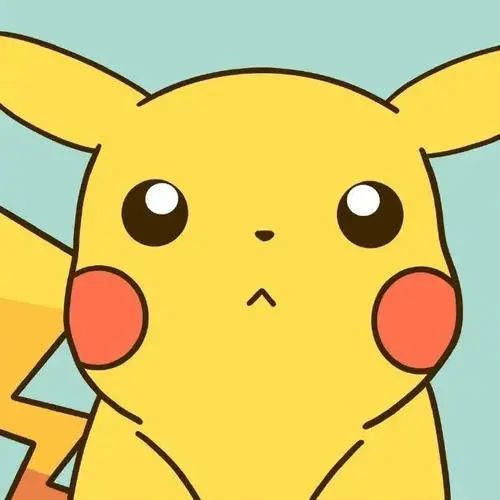
Empowering Relational Network by Self-Attention Augmented Conditional Random Fields for Group Activity Recognition(eccv 2020)
核心思想
网络结构
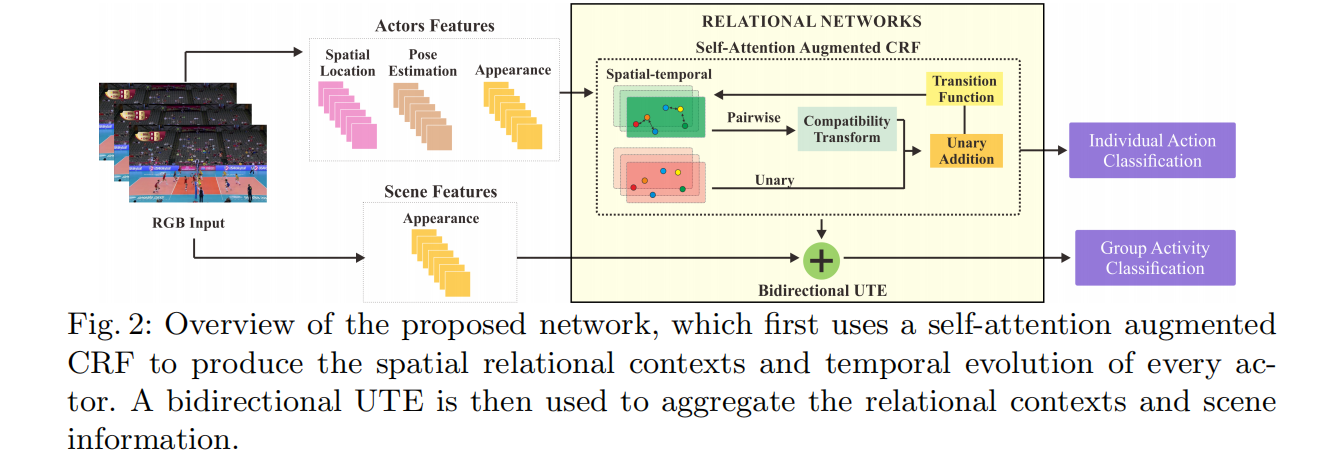
整体的网络可以分为两个阶段,第一阶段为特征提取网络,第二阶段为关系推理网络。appearence信息是通过faster rcnn+I3D得到的。其中,先使用faster rcnn得到检测框信息(它是在用预训练的coco模型在volleyball数据集上进行微调得到的)再用I3D提取特征,在这个过程中需要用到检测框信息(I3D是在预训练的kinetics模型在volleyball数据集上进行微调得到的)。场景信息即直接用I3D处理得到的视频特征。
创新模块
temporal and spatial self-attention
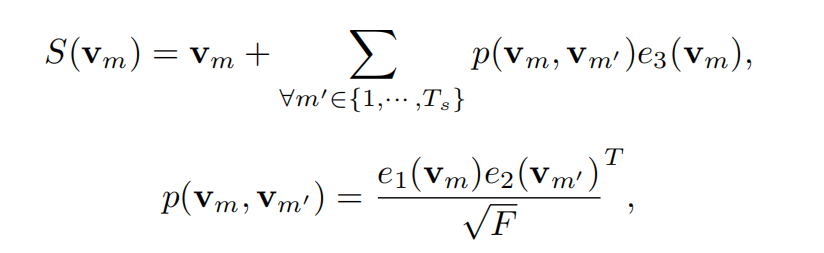

空间注意力,尝试将整个图划分为不同大小的子图,对于不同规模的子图,计算其特征,对于这些进行加权平均,权重是可学习的。
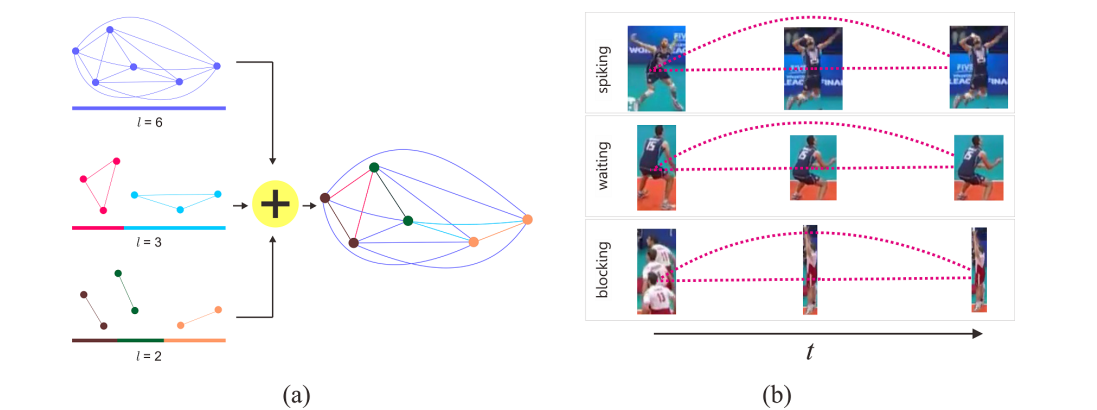
mean-field inference algorithm
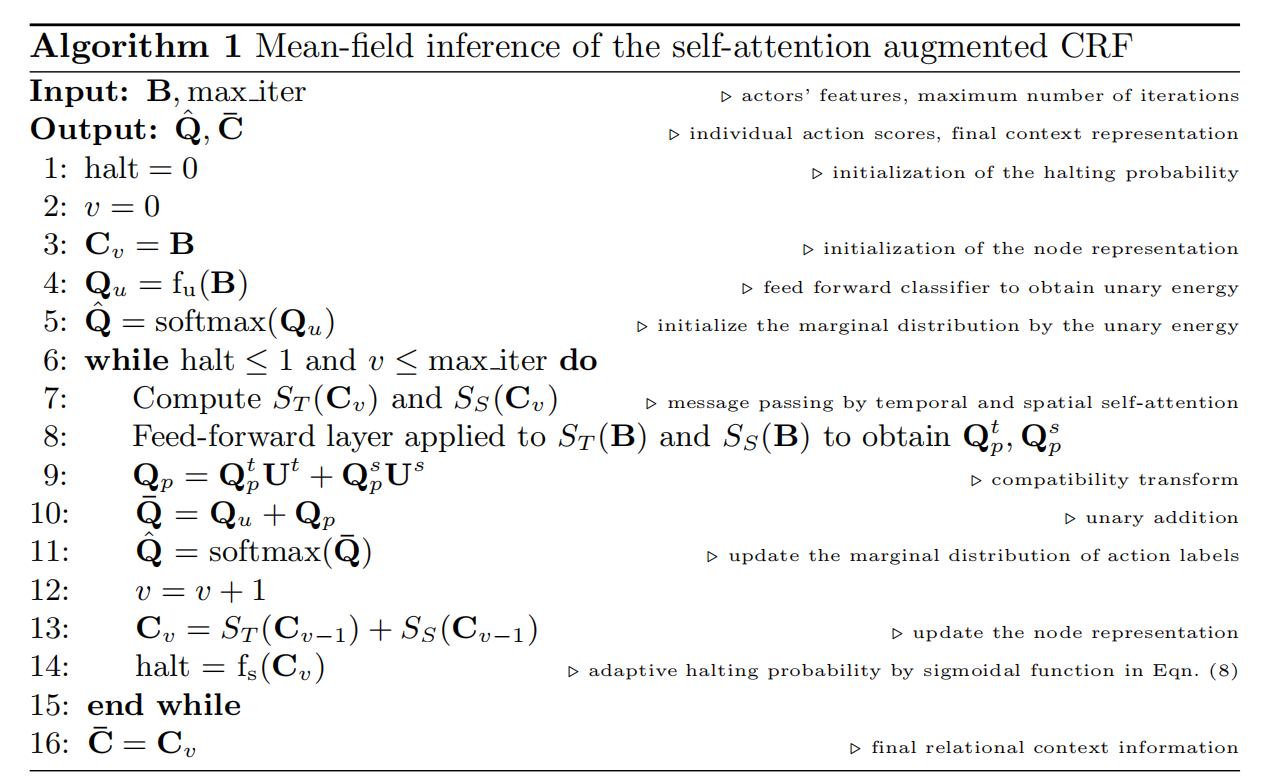
简单地用图示来表示算法过程:
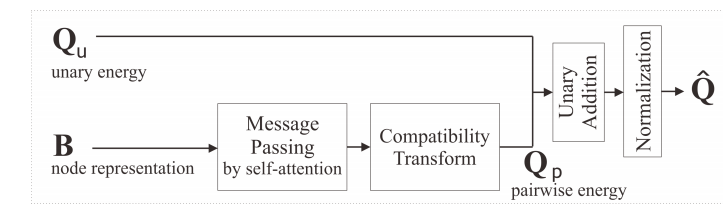
bidirectional universal transformer encoder (UTE)
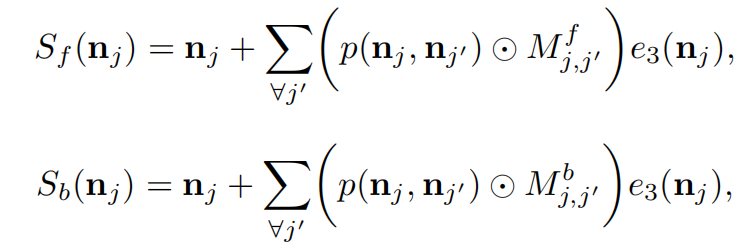

- Post title:论文阅读笔记:“Empowering Relational Network by Self-Attention Augmented Conditional Random Fields for Group Activity Recognition(eccv 2020)”
- Post author:sixwalter
- Create time:2023-08-05 11:14:26
- Post link:https://coelien.github.io/2023/08/05/paper-reading/paper_reading_059/
- Copyright Notice:All articles in this blog are licensed under BY-NC-SA unless stating additionally.
Comments