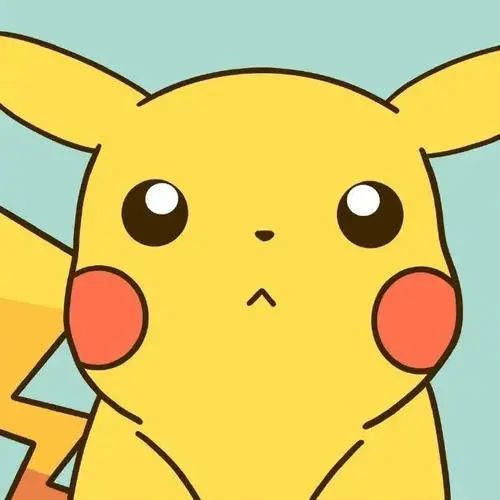
facebook PySlowFast 安装
环境配置并处理依赖
docker环境配置方法参考docker for pytorch.md
下面是项目安装所需要的依赖,详见该仓库
fvcore
轻量级的核心库,提供视觉框架开发最通用和基础的功能
Features:
Besides some basic utilities, fvcore includes the following features:
- Common pytorch layers, functions and losses in fvcore.nn.
- A hierarchical per-operator flop counting tool: see this note for details.
- Recursive parameter counting: see API doc.
- Recompute BatchNorm population statistics: see its API doc.
- A stateless, scale-invariant hyperparameter scheduler: see its API doc.
FFmpeg
用来处理多媒体内容(音频,视频,字幕)的工具库集合。 官方网站:website
sth interesting: Using Git to develop FFmpeg
Tools
- ffmpeg is a command line toolbox to manipulate, convert and stream multimedia content.
- ffplay is a minimalistic multimedia player.
- ffprobe is a simple analysis tool to inspect multimedia content.
- Additional small tools such as
aviocat
,ismindex
andqt-faststart
.
PyAV
是对FFmpeg python 风格的binding,提供对下层的库提供强大的控制。PyAV 用于通过容器、流、数据包、编解码器和帧直接和精确地访问媒体。文档链接
psutil
获取运行进程和系统利用率信息的库(CPU, memory, disks, network, sensors)。文档链接
tensorboard
PyTorchVideo
PyTorchVideo is a deeplearning library with a focus on video understanding work. PytorchVideo provides reusable, modular and efficient components needed to accelerate the video understanding research. PyTorchVideo is developed using PyTorch and supports different deeplearning video components like video models, video datasets, and video-specific transforms.
Detectron2
https://github.com/facebookresearch/detectron2
pytorch
install from binaries
- PyTorch is supported on Linux distributions that use glibc >= v2.17
- Ubuntu, minimum version 13.04
- Python 3.7 or greater
building from source
install bleeding edge PyTorch code
pre-knowledge
GPU 计算能力表:https://developer.nvidia.com/cuda-gpus

CUDA是一个工具包,是NVIDIA推出的用于自家GPU上的并行计算框架。For convenience, the NVIDIA driver is installed as part of the CUDA Toolkit installation
cuDNN是一个SDK,是一个专门用于神经网络的加速包,注意,它跟我们的CUDA没有一一对应的关系,即每一个版本的CUDA可能有好几个版本的cuDNN与之对应,但一般有一个最新版本的cuDNN版本与CUDA对应更好,链接地址
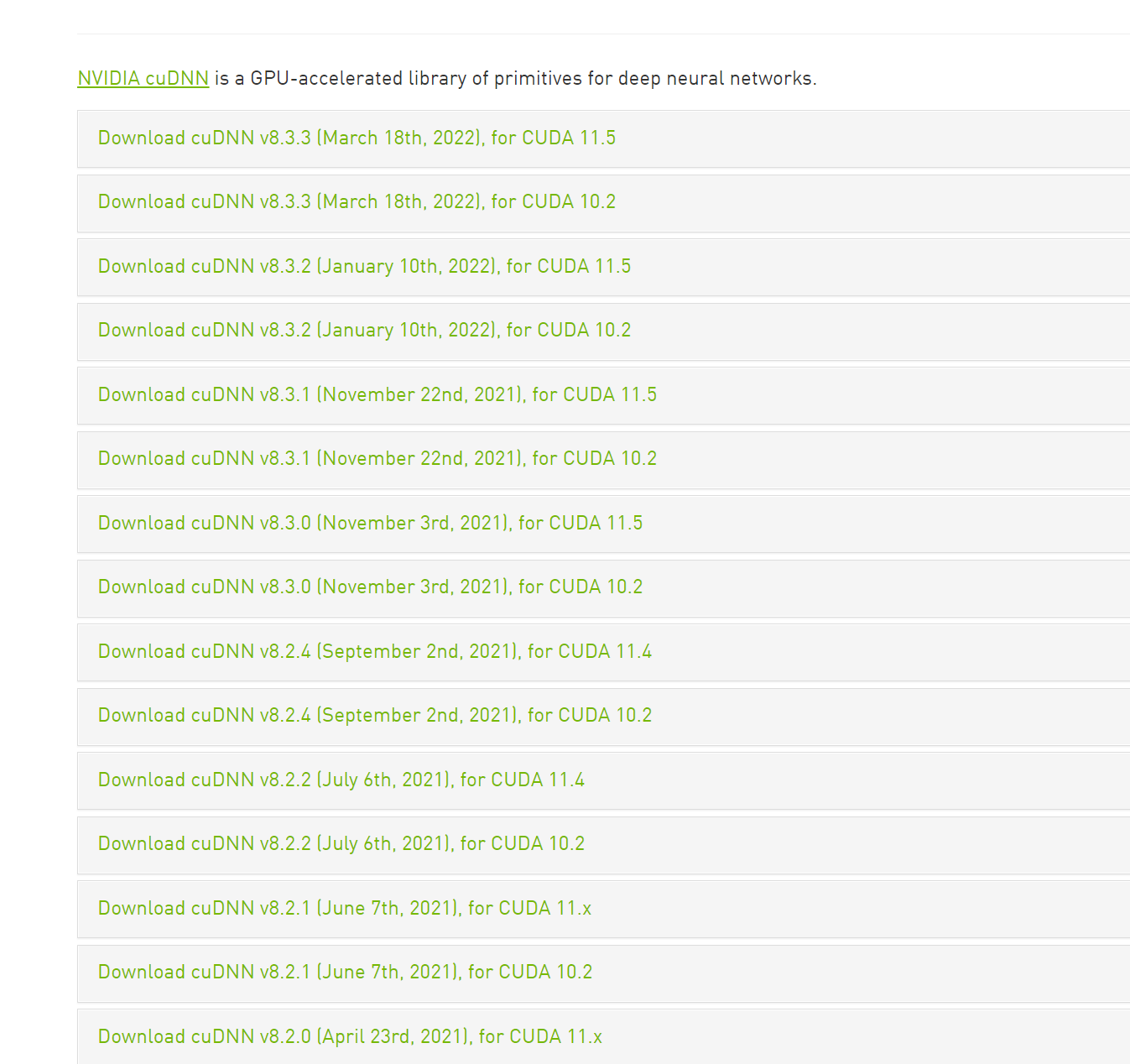
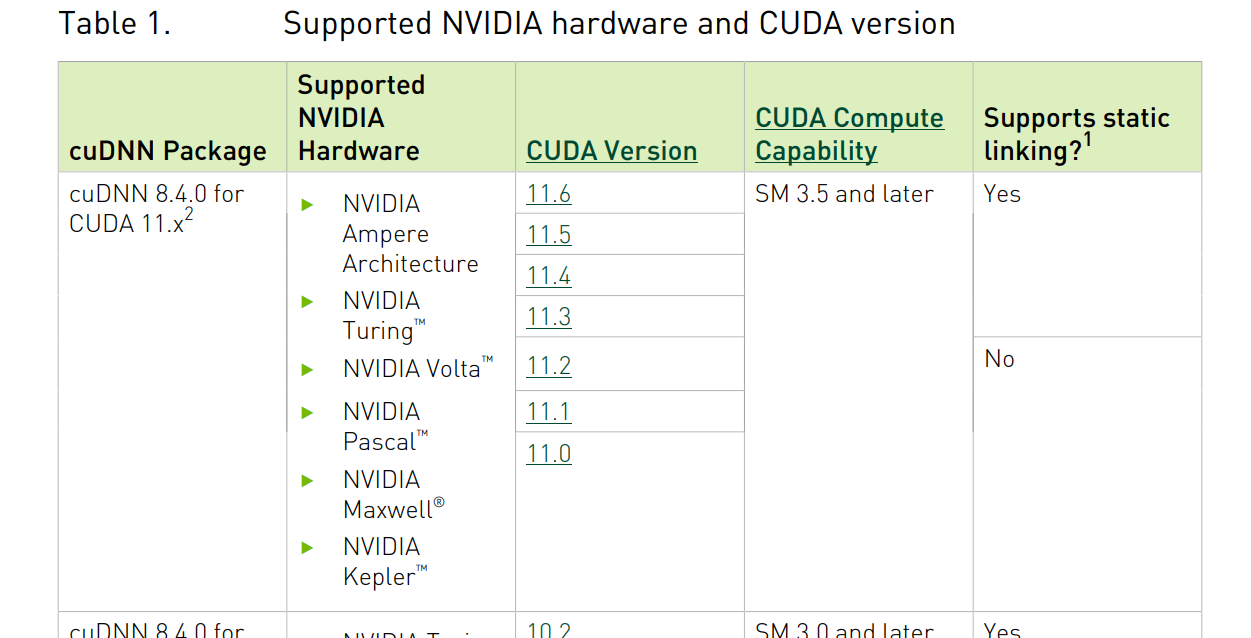
数据下载与处理
kinetics数据集下载 github:https://github.com/cvdfoundation/kinetics-dataset
- 我下载的数据集为kinetics-700-2020,可参考:https://github.com/cvdfoundation/kinetics-dataset#kinetics-700-2020
- 数据集下载:bash ./k700_2020_downloader.sh
- 确定下载目录和数据集目录
- 将训练,验证,测试数据集,标注下载到对应目录
- 使用命令:wget -P 下载到指定目录 -c 断点续传 -i 下载多个文件
- 数据集处理:bash ./k700_2020_extractor.sh
- 分别解压训练,验证,测试数据集和标注
- 使用命令 tar zxf 来解压 -C 解压到的目录
下载数据集也可参考https://github.com/activitynet/ActivityNet/tree/master/Crawler/Kinetics,但只有k600之前的
可参考该仓库进行数据集准备:https://github.com/facebookresearch/video-nonlocal-net/blob/main/DATASET.md
获得类别标签映射,因为原仓库是针对k400的,所以我重新生成了映射
详见data_process文件夹下的preprocess.py的generatejsonmap函数
使用gen_py_list.py改变文件夹名称,并对训练集和验证集生成txt列表.
若gen_py_list.py中途报错,则生成的txt列表会不全,这里写了个简单的函数来进行重新生成(preprocess.py下的generatetxtlist())。若输出与实际数据集视频数相等,则生成正确( 即和“ls -lR /tmp/data/train | grep “^-“ | wc -l ”的结果进行比较)
使用downscale_video_joblib.py把视频的高度缩小为256 pixels
检查文件数是否匹配即可
调整视频大小到256, 准备训练集,验证集,测试集的csv文件如
train.csv
,val.csv
,test.csv
. 格式如下:1
2
3
4
5path_to_video_1 label_1
path_to_video_2 label_2
path_to_video_3 label_3
...
path_to_video_N label_N依据txt列表生成CSV文件:
1 | # data_process/preprocess.py |
- Post title:docker内配置facebook pyslowfast环境
- Post author:sixwalter
- Create time:2023-08-05 11:14:26
- Post link:https://coelien.github.io/2023/08/05/projects/kinetics project/installation of slowfast/
- Copyright Notice:All articles in this blog are licensed under BY-NC-SA unless stating additionally.